Your Cloud Bill Is Too High—Here’s Why On-device AI Compute Wins
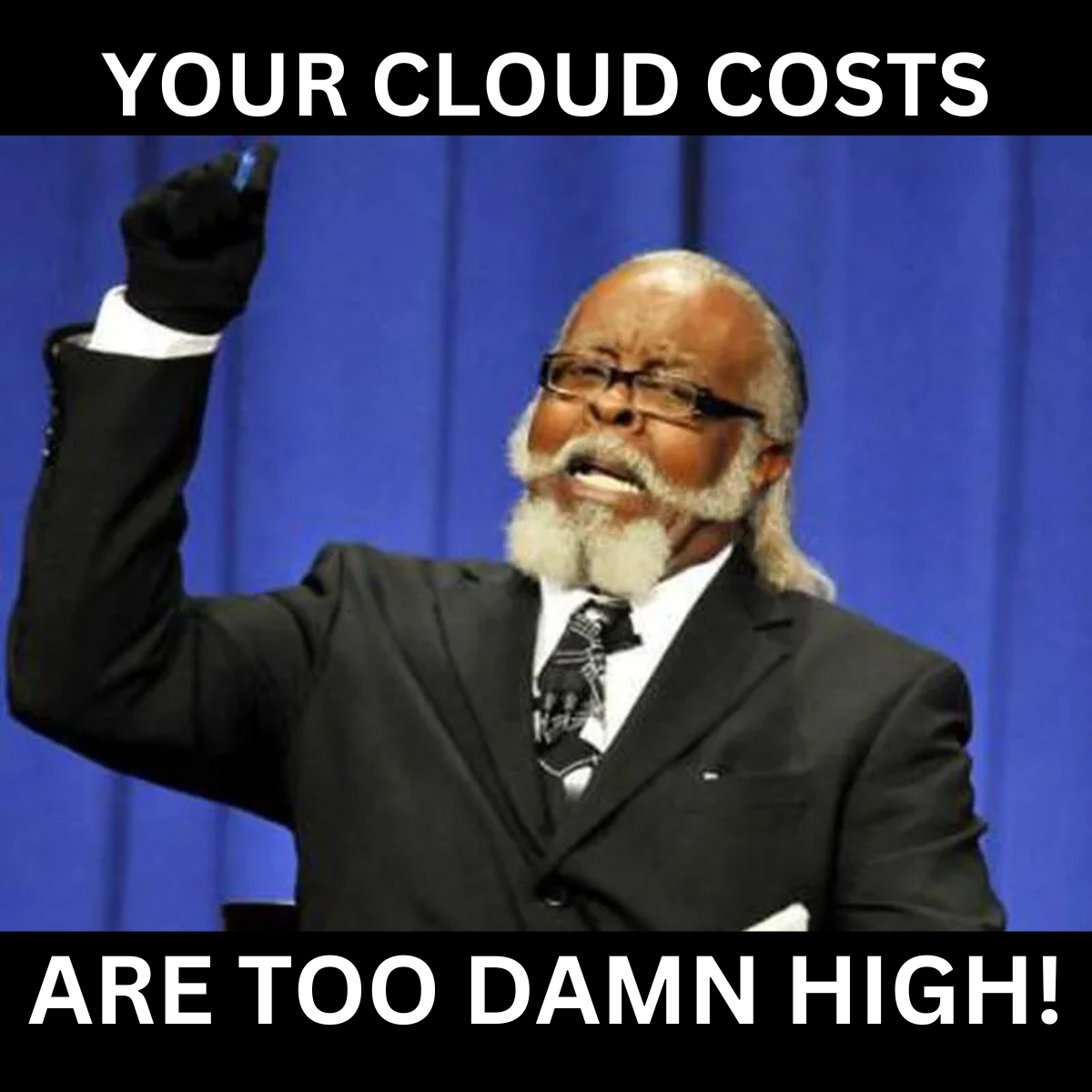
Comparing Cloud and On-device Compute
“A recent poll showed that about 80% of all smartphone users only use between 8 and 12 apps on a consistent basis, typically for tasks like email, messaging, social networking, news, games, weather, and photography. But only about 10% to 15% of users said they also use smartphones for serious productivity.”
This suggests that while the breadth of applications has remained stable, the power of the devices running them has changed dramatically. While ChatGPT has significantly improved the productivity aspect of life, the other 80% of time spent on smartphones has not yet been boosted by AI.
Over the past decade, smartphone performance has undergone an exponential transformation. Based on publicly available Geekbench scores and similar benchmarks from 2014 to 2024, top-tier smartphone processing power has increased roughly by a factor of 20 to 25. In early 2014, flagship devices often scored around 40,000–50,000 on AnTuTu. Fast forward to 2023–2024, and flagship devices routinely exceed 1,000,000 on the same benchmark, with some models even hitting 1.3 million.
To put this growth into perspective, let’s compare two reference points with Geekbench:
Historical Baseline: iPhone 6 (A8, 2014)
- Geekbench 3 (circa 2014–2015) single-core: ~1,500–1,600
- Geekbench 4 single-core: ~1,400
- Geekbench 5 single-core: ~300
The iPhone 6 was considered a powerful device at the time, but as benchmarks evolved, its performance in absolute terms appears significantly lower when measured with modern Geekbench versions.
Modern Reference: iPhone 15 Pro (A17 Pro, late 2023)
- Geekbench 6 single-core: ~2,900–3,000
- Multi-core: ~7,000–7,500
This represents a staggering 20–25× improvement in just ten years. If we translate this into a Compound Annual Growth Rate (CAGR), compute power in smartphones increases by approximately 38% yearly.
Assuming this trend keeps on following on growing with the advent of AI. We are missing a compute growth of 37% faster than overall growth of Public Cloud. Hence the cost per unit of compute on edge will fall faster than cloud prices. It makes sense to invest long term in on-device as a part of compute strategy.
The Shift in Compute Economics
Now, let’s compare this growth to cloud computing. Public cloud infrastructure has certainly advanced, but its growth in raw compute capability has not kept pace with on-device performance. While cloud providers continue to scale, their CAGR remains significantly lower—meaning the cost per unit of compute on the edge (smartphones and other devices) is decreasing much faster than cloud computing prices.
With the rise of AI-driven applications, this trend is even more pronounced. AI models that previously required massive cloud clusters can now run efficiently on high-end smartphones. Cloud computing will always have its place, especially for storage, data aggregation, and intensive processing tasks, but relying on cloud alone for user-facing computation is becoming increasingly expensive.
The key takeaway? The cost efficiency of on-device compute is improving at a faster rate than cloud compute, and businesses that adapt their strategies accordingly will have a significant competitive advantage.
Impact of On-device Compute in the U.S.
In the U.S., the growth of smartphone computing power follows a similar trajectory to global trends. Currently, the estimated cloud computing capacity of AWS in the U.S. is around 500 million vCPUs. Meanwhile, with approximately 310 million smartphones in the country—each with an average Geekbench score equivalent to 8 vCPUs (double that of India)—on-device computing power is becoming a formidable alternative.
A typical modern smartphone in the U.S., spanning both iOS and Android from midrange to flagship devices, scores between 1,000 and 1,300 in single-core performance and 3,000 to 4,000 in multi-core on Geekbench 6. This translates into a total on-device compute capacity of approximately 1.2 billion vCPUs—equivalent to the entire U.S. cloud computing capacity but growing 25% faster year over year.
To put this into perspective for a real-world application, consider an app like Etsy with 30 million monthly active users (MAU), each engaging in 10 sessions per month with an average session length of two minutes. This results in a total monthly compute requirement of:
- 600 million minutes of 8 vCPUs
- Equivalent to 800 million vCPU hours
- Cloud compute cost: ~$10 million per month or $120 million per year
This means that the potential compute power available on-device for an app like Etsy is nearly equal to the total cloud compute capacity, offering a significant opportunity for cost savings and efficiency improvements.
Furthermore, the price-performance gap between cloud and on-device compute is widening. The cost per unit of on-device compute is becoming increasingly competitive, with on-device processing costs decreasing at a rate of over 25% YoY, compared to cloud compute growth at just 16% YoY. This trend mirrors the broader infrastructure shift seen in other industries, such as electricity demand in the U.S. The official nationwide forecast for electricity demand recently jumped from a 2.8% to an 8.2% growth rate over the next five years, reaching 66 GW by 2029, with preliminary updates suggesting an even steeper rise of 15.8%.
The takeaway is clear: as on-device compute continue to advance at an accelerated pace, companies that rely heavily on cloud infrastructure must reassess their strategies. Leveraging on-device compute can significantly reduce costs, improve efficiency, and position businesses to take advantage of a rapidly evolving technological landscape.
Impact of On-device Compute in India
With on-device computing power growing at a significantly faster rate than cloud infrastructure, the implications vary across different markets. In regions like the U.S., where cloud adoption has been deeply ingrained for years, the shift toward on-device compute is driven by performance optimizations and cost savings. However, in a country like India, the dynamics are different.
India has always approached technology from a scale-first perspective, optimizing for billions of users with cost efficiency as a primary constraint. The country leapfrogged the desktop computing era, embracing mobile-first digital transformation at an unprecedented pace. This means that the potential for on-device computing in India is not just an opportunity—it’s a necessity. Given the sheer volume of smartphone users and the rapid evolution of affordable yet powerful devices, the case for shifting compute workloads from the cloud to the edge is even more compelling.
Let’s look at viability and timing to decide how ready is on-device for you. We will look at the base of where each stands today in India.
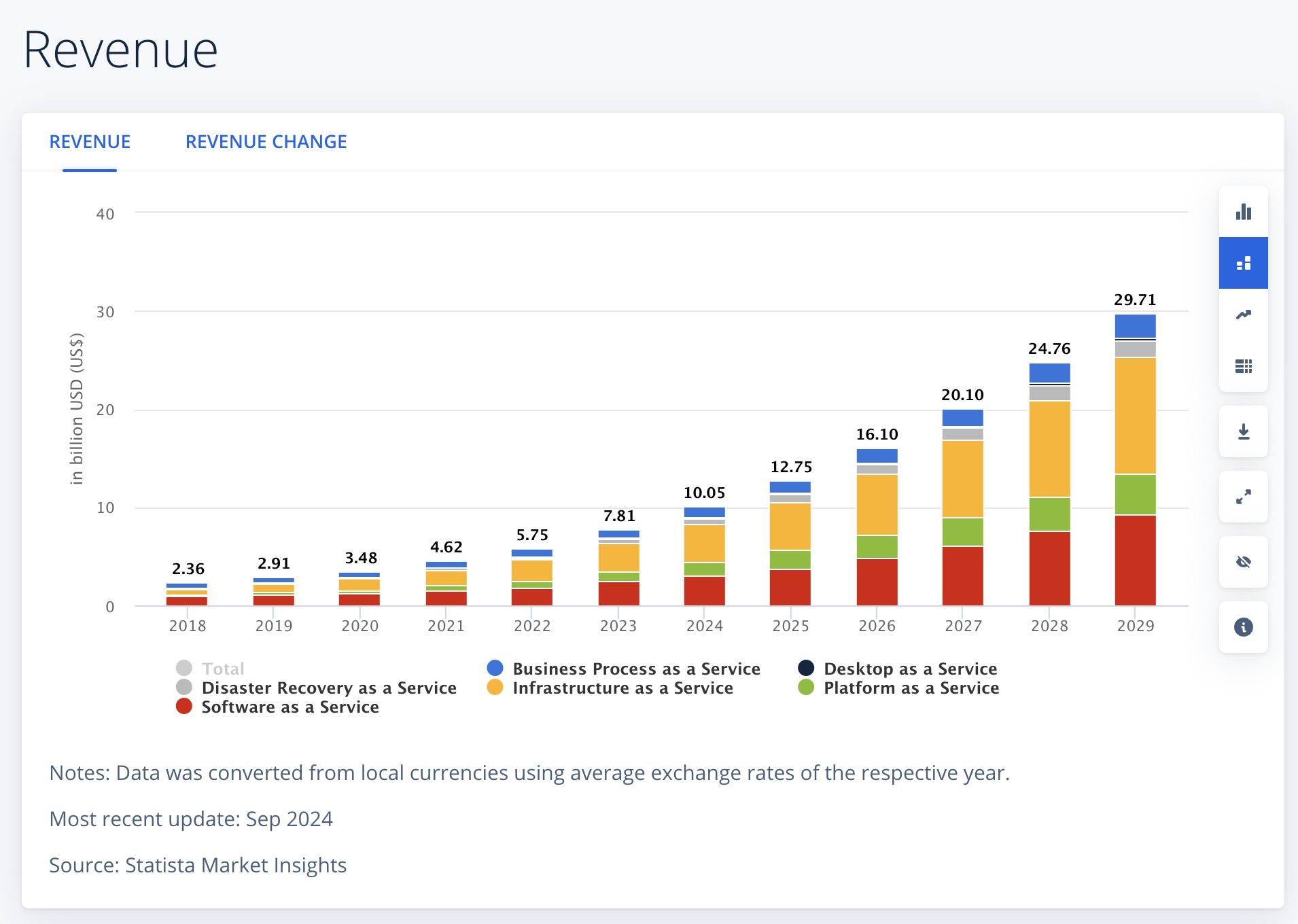
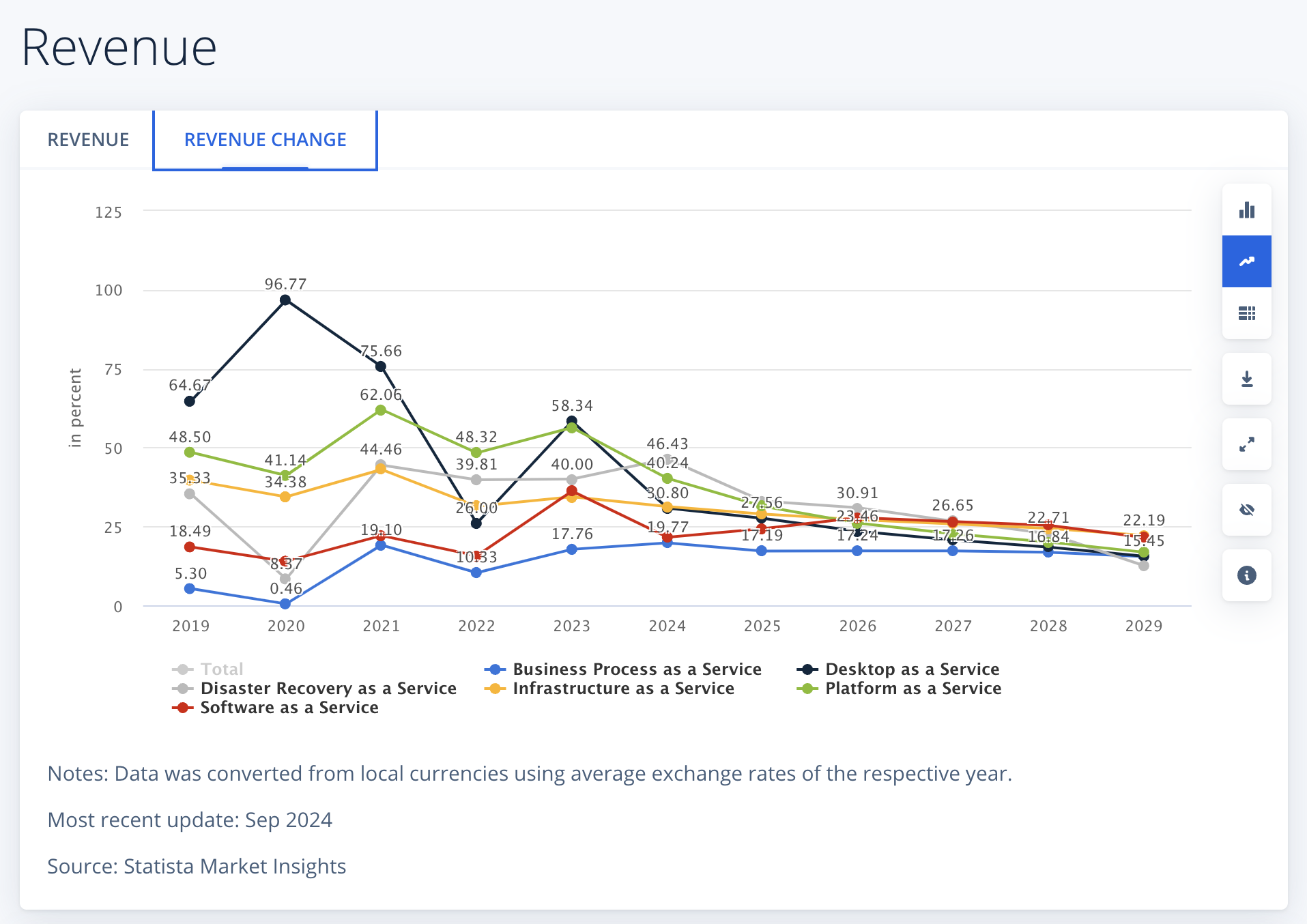
The Public Cloud Market in India is projected to grow to ~$30B by the year 2029 at CAGR ~23%. There is a fascinating compute source available in the hands of users which scales decentralized rather than via enterprise capital. Let’s compare cloud computing with the on-device compute capabilities available in India. Fortunately, India has the best–as well as the cheapest–smartphones in the market, meaning there is a wide variety of smartphone compute capacities as well.
AWS India, by back-of-the-envelope estimates, comes to 50-70M vCPUs. This means that the total cloud compute capacity in India is about ~240M vCPUs (assuming AWS at 33% market share).
There are 700M smartphones in India with average 4 core CPU -> 1.8B vCPUs in cloud terms! This is NINE TIMES the Indian cloud capacity.
“A New mid-range smartphone in India: ~500–700 single-core / 1,500–2,000 multi-core on Geekbench 6.”
To put numbers into perspective for mobile apps:
- For an App with 60M MAU, 10 sessions/month and 2 min session, you have monthly compute worth 1.2B mins of 4 vCPUs amounting = $0.05*1.2B/60 = ~$5M/month.
That’s ~$60M/year – a massive number considering the overall cloud bill is ~$100M for the best of the best.
This shows us that on-device compute presents a massive cost-saving opportunity for mobile apps. Even after factoring in availability constraints, leveraging on-device processing could reduce cloud costs by more than 60%—a significant efficiency gain. Additionally, the price-performance gap between cloud and edge computing is widening, with on-device compute becoming more cost-effective at a rate of over 15% YoY. This trend suggests that companies heavily reliant on cloud infrastructure today should seriously reconsider their compute strategy, integrating more on-device processing to optimize costs and scalability.
The Future of Compute: Balancing Cloud and On-device Processing
While on-device compute presents a compelling opportunity for cost savings and performance improvements, not everything can or should be processed on the edge. Aggregation, large-scale data analysis, and certain centralized services will always require cloud infrastructure. However, for user-focused compute—real-time interactions, personalized AI processing, and latency-sensitive workloads—on-device computing is not just viable but increasingly essential for scalability and cost efficiency.
As we’ve seen, the economics of computing are shifting. The rapid growth of smartphone processing power has outpaced that of cloud infrastructure, making on-device compute an increasingly attractive alternative. In India, where technology is optimized for scale and cost, tapping into the vast decentralized compute power of smartphones could unlock significant efficiencies. In the U.S., where on-device compute capacity is nearly equal to total cloud capacity and growing faster, the opportunity to offload workloads from the cloud to edge devices is even more apparent.
Beyond cost savings, this shift represents a fundamental change in how applications are built and optimized. Companies that embrace on-device compute can not only reduce their cloud expenditures but also enhance user experiences by reducing latency and improving personalization. Moreover, with AI continuing to advance, leveraging edge compute can drive new revenue opportunities by unlocking deeper insights into user behavior.
For more on this topic, check out this post about how on-device AI can unlock new insights about users in order to generate net new revenue and reduce the humongous 50% drop off rates across apps. The future of computing isn’t about replacing the cloud—it’s about balancing cloud and edge to create more efficient, scalable, and cost-effective applications.